Predictive Maintenance
Predictive maintenance is a proactive maintenance strategy that uses data and analytics to optimize maintenance schedules, reduce costs, and improve equipment performance. By leveraging real-time data from sensors, machine logs, and other sources, organizations can identify potential issues before they become more significant problems. With predictive maintenance, we not only provide an exact status of the systems installed in the plant, we are also able to predict the ideal maintenance time using our AI model.
- 9Optimized Maintenance Schedules
- 9Real-Time System Status & Predictions with AI
- 9Priority-Based Maintenance Alerts
- 9Detailed Asset Data & History
- 9Downtime Tracking & Insights
- 9AI-Driven Anomaly Detection
Optimal Maintenance Timing
Our system not only predicts the optimal maintenance timing for your equipment components but also provides clear maintenance priorities. Thanks to a wear indicator, you can instantly see which parts need special attention, allowing you to efficiently plan maintenance actions and prevent unplanned downtime.
Downtime Monitoring
With our downtime monitoring, error times for each unit are summed up and clearly displayed for the desired period. This allows for quick identification of idle times, and any problematic equipment components become immediately visible. This enables you to take targeted action and minimize the risk of failures.
Asset Insights
Our solution offers comprehensive asset details, including usage data and maintenance history.
These clear and concise insights are displayed in historical and graphical formats, so you always have an overview of the condition and performance of your assets, enabling you to make informed decisions.
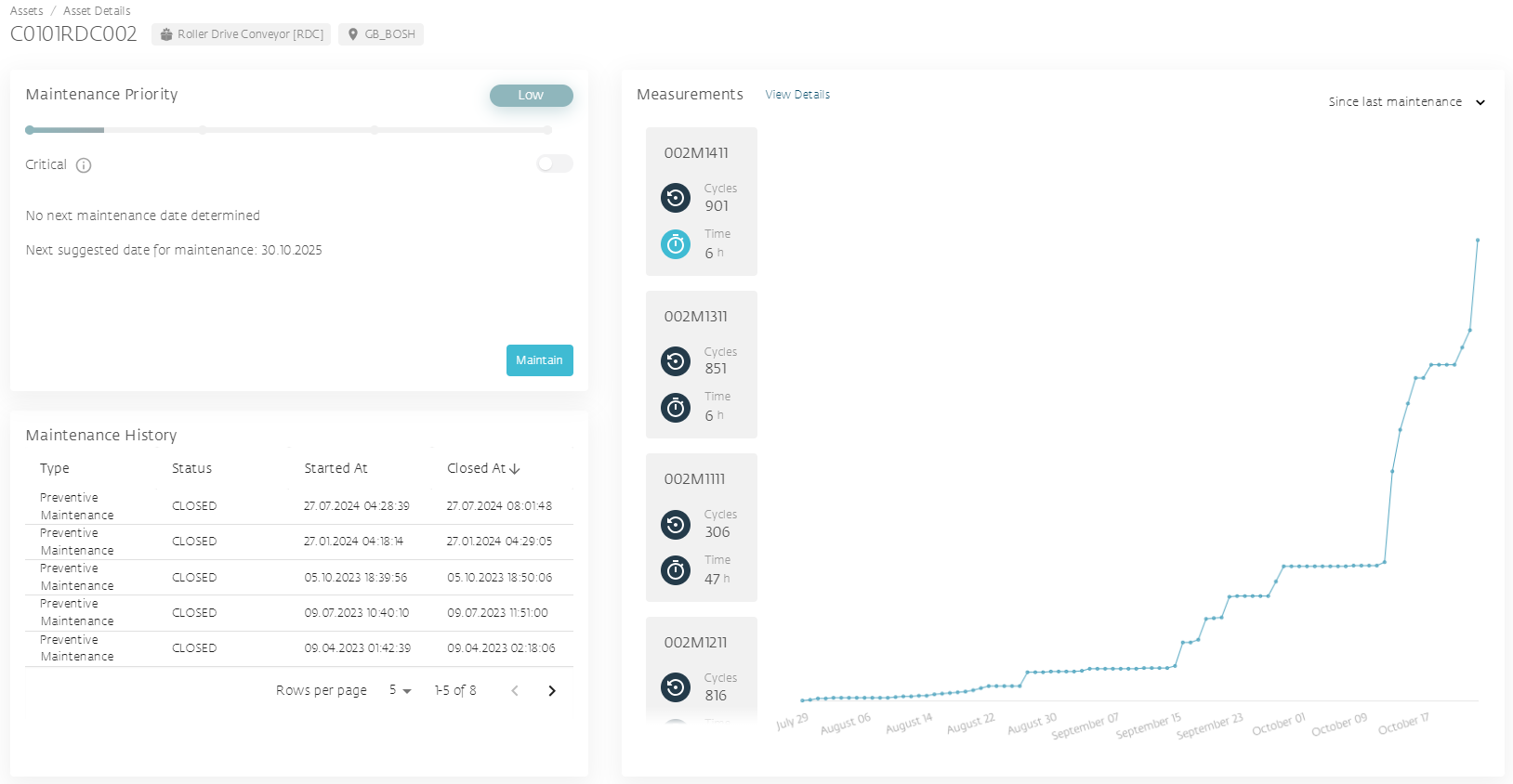
Anomaly Detection
Our anomaly detection identifies unusual usage patterns in your equipment. The system detects irregularities early and alerts you when components deviate from normal operation. This allows potential issues to be addressed promptly and unexpected failures to be avoided.